How AI & Big Data Are Revolutionizing Insurance
AI-Powered Risk Assessment
AI and big data are transforming how insurance companies assess and manage risk. Traditional methods often relied on broad demographic data and historical averages, leading to potentially inaccurate and unfair premiums. AI, however, offers a more nuanced and personalized approach, leveraging vast datasets and sophisticated algorithms to create more accurate risk profiles. This allows insurers to offer more competitive and equitable pricing while also mitigating their own risk exposure.
AI algorithms analyze diverse data sources, going beyond traditional actuarial methods to provide a more comprehensive understanding of risk. This includes not only demographic information but also real-time data streams, enabling dynamic risk assessment and pricing.
AI-Driven Driver Behavior Analysis for Car Insurance
Imagine a scenario where a young driver, Sarah, uses a telematics device installed in her car. This device collects data on her driving habits, such as speed, acceleration, braking patterns, time of day driving, and even location. An AI algorithm analyzes this data, comparing Sarah’s driving behavior to a vast database of other drivers. The algorithm identifies patterns and anomalies, such as frequent hard braking or speeding in high-risk areas. Based on this analysis, the AI system can generate a personalized risk score for Sarah, which then informs her car insurance premium. If Sarah consistently demonstrates safe driving habits, her premium might be lower than the average for her age group. Conversely, if the AI detects risky behavior, her premium might be adjusted accordingly, incentivizing safer driving practices. This dynamic pricing model offers a fairer and more personalized approach compared to traditional methods that primarily rely on age and location.
Comparison of Traditional and AI-Driven Risk Assessment
Traditional actuarial methods rely heavily on historical data and statistical models. These methods group individuals into broad categories based on factors like age, location, and driving history. While effective in providing general risk estimates, they often fail to capture the nuances of individual behavior. AI-driven risk assessment, on the other hand, uses machine learning algorithms to analyze a much wider range of data points, including real-time driving behavior, claims history, and even social media activity (with appropriate privacy considerations). This granular level of analysis allows for more accurate and personalized risk assessments. The AI approach can identify subtle patterns and correlations that traditional methods might miss, leading to more precise pricing and risk management. For example, while traditional methods might charge a higher premium for all young drivers, AI can differentiate between young drivers who are consistently safe and those who exhibit risky behavior, resulting in fairer premiums.
Ethical Implications of AI in Risk Profiling
The use of AI in insurance risk profiling raises several ethical concerns. One key issue is bias. If the training data used to develop the AI algorithms contains biases, the resulting risk assessments may unfairly discriminate against certain groups. For example, if the data reflects historical biases in traffic enforcement, the AI might unfairly penalize drivers from certain demographic groups. Another concern is data privacy. The collection and use of large amounts of personal data raise questions about transparency and consent. Insurers must ensure that data collection and usage practices are transparent and comply with all relevant privacy regulations. Finally, the “black box” nature of some AI algorithms can make it difficult to understand how risk assessments are made, leading to a lack of accountability and potential for unfair treatment. Addressing these ethical concerns requires careful consideration of data sources, algorithm design, and regulatory oversight to ensure fairness and transparency in AI-driven risk assessment.
Fraud Detection and Prevention
The insurance industry faces significant losses due to fraudulent claims. The sheer volume of claims processed daily makes manual detection incredibly difficult and inefficient. However, the application of machine learning (ML) algorithms offers a powerful solution, enabling insurers to identify and prevent fraudulent activities with greater accuracy and speed than ever before. These algorithms excel at identifying subtle patterns and anomalies that might escape human scrutiny.
Machine learning algorithms can identify patterns indicative of insurance fraud by analyzing vast datasets containing policyholder information, claim details, and external data sources. These algorithms employ various techniques, including anomaly detection, classification, and regression, to pinpoint suspicious activities. For instance, anomaly detection algorithms can identify claims that deviate significantly from the norm, while classification algorithms can predict the likelihood of a claim being fraudulent based on various risk factors. The use of external data, such as social media activity or public records, further enhances the accuracy of these predictions.
AI-Powered Fraud Detection System Workflow
The following flowchart illustrates the typical steps involved in an AI-powered fraud detection system:
[Imagine a flowchart here. The flowchart would begin with “Claim Submission,” branching to “Data Collection and Preprocessing” (gathering claim details, policyholder information, external data). This would then feed into “Feature Engineering” (creating relevant variables for the model). Next would be “Model Training” (using historical data to train a machine learning model, for example, a Random Forest or a Neural Network). The trained model would then process incoming claims in “Fraud Prediction,” outputting a risk score. A high risk score would trigger “Manual Review” by a claims adjuster. Claims with low risk scores would be processed automatically in “Claim Processing.” Finally, the entire process would feed back into “Model Refinement” to continuously improve the accuracy of the system.]
Examples of Insurance Fraud Detected by AI
AI is particularly effective at detecting several types of insurance fraud. For example, staged accidents, where individuals deliberately cause collisions to file fraudulent claims, often exhibit patterns in the timing, location, and severity of accidents that ML algorithms can easily identify. Similarly, AI can detect patterns of organized fraud rings, where multiple individuals collude to submit false claims. The algorithms can identify connections between seemingly unrelated claims based on shared characteristics such as addresses, phone numbers, or even social media connections. Another common type of fraud, inflated claims, where individuals exaggerate the extent of damage or losses, can also be detected by comparing claim amounts to industry benchmarks and historical data. AI can also detect instances of identity theft used to file fraudulent claims by analyzing inconsistencies in provided personal information against known databases. For instance, discrepancies between the provided address and the location of the accident could trigger a deeper investigation. In a real-world example, a major US insurer reported a 20% reduction in fraudulent claims after implementing an AI-powered fraud detection system. This resulted in significant cost savings and improved efficiency in claim processing.
Personalized Insurance Products
The ability to tailor insurance products to individual customer needs is rapidly transforming the insurance industry. Big data and AI are the driving forces behind this revolution, allowing insurers to move beyond one-size-fits-all policies and offer more relevant, affordable, and attractive coverage options. This personalized approach enhances customer satisfaction, increases policy retention, and ultimately boosts profitability for insurance providers.
Big data analytics provides insurers with an unprecedented understanding of customer behavior, preferences, and risk profiles. By analyzing vast datasets encompassing demographics, lifestyle choices, driving habits (in the case of auto insurance), health records (for health insurance), and purchasing patterns, insurers can identify distinct customer segments with shared characteristics and tailor insurance offerings accordingly. This granular level of understanding allows for the creation of truly personalized products that resonate with specific customer groups.
Personalized Product Examples
Three distinct personalized insurance products, designed using big data analysis, are Artikeld below. These examples demonstrate the potential of data-driven product development in the insurance sector.
Product A: “SmartDriver” Auto Insurance | Product B: “WellnessPlus” Health Insurance | Product C: “HomeSafe” Homeowners Insurance |
---|---|---|
Targeted at young, responsible drivers with a proven history of safe driving (demonstrated through telematics data). Offers lower premiums based on driving behavior and usage-based insurance (UBI) programs. Includes features such as accident forgiveness and roadside assistance. | Targeted at health-conscious individuals who actively engage in preventative healthcare. Offers lower premiums for participation in wellness programs (e.g., gym memberships, regular check-ups). Includes benefits such as telehealth consultations and discounts on health-related products. | Targeted at homeowners who implement smart home security systems. Offers discounted premiums for installation and usage of smart home devices (e.g., security cameras, smoke detectors). Includes features such as emergency home repair services and replacement cost coverage. |
Big Data and Customer Understanding
Big data plays a crucial role in understanding customer preferences and needs. By analyzing vast datasets, insurers can identify patterns and trends that inform product development. For example, analyzing social media activity can reveal customer concerns about specific insurance coverage gaps, while analyzing claims data can highlight areas where product improvements are needed. This data-driven approach ensures that new products are not only innovative but also address real customer needs, leading to higher customer satisfaction and improved retention rates.
AI-Powered Personalized Communication
AI is used extensively to personalize customer communication and marketing efforts. Chatbots powered by natural language processing (NLP) can provide instant customer support and answer policy-related questions. AI algorithms can analyze customer data to tailor marketing messages and offers, ensuring that each customer receives relevant and timely communication. For example, an insurer might use AI to send targeted email campaigns promoting a specific product based on a customer’s demographics, risk profile, and past interactions. This personalized approach improves customer engagement and increases the effectiveness of marketing campaigns.
Claims Processing and Automation
The insurance industry is ripe for disruption, and AI and robotic process automation (RPA) are leading the charge, particularly in claims processing. Automating this traditionally labor-intensive process offers significant improvements in efficiency, cost-effectiveness, and accuracy. By leveraging AI’s capabilities, insurers can streamline operations, enhance customer satisfaction, and gain a competitive edge.
Claims processing, traditionally a manual and time-consuming task, involves several stages from initial claim submission to final settlement. Automating this workflow using AI and RPA dramatically reduces processing time and human error, leading to faster payouts and improved customer experience.
Automated Claims Processing Workflow
The implementation of AI and RPA in claims processing significantly streamlines the workflow. The process typically begins with the claimant submitting a claim, often digitally. This claim data is then automatically extracted and validated using OCR (Optical Character Recognition) and NLP (Natural Language Processing) technologies. RPA bots then take over, routing the claim to the appropriate department, initiating the verification process (e.g., checking policy details, contacting witnesses), and even scheduling assessments if necessary. AI algorithms analyze the claim data, identifying potential fraud or inconsistencies, and flagging them for further investigation. Finally, once all checks are complete, the system automatically processes the payment. Throughout the process, AI continuously learns and improves its accuracy, reducing the need for human intervention.
Efficiency and Cost-Effectiveness of Automated Claims Processing
Automated claims processing offers substantial advantages over manual methods. Manual processing is prone to errors, delays, and high administrative costs. For example, a study by [Insert credible source and study details here, e.g., a consulting firm’s report] found that automating claims processing reduced processing time by an average of X% and decreased operational costs by Y%. The reduced processing time leads to faster claim settlements, enhancing customer satisfaction and loyalty. The cost savings stem from reduced labor costs, fewer errors requiring correction, and decreased administrative overhead. Furthermore, automated systems can handle a much larger volume of claims simultaneously compared to manual processing, allowing insurers to scale their operations efficiently.
AI-Enhanced Claims Assessment Accuracy and Speed
AI significantly improves the accuracy and speed of claims assessments. Traditional methods often rely heavily on human judgment, which can be subjective and prone to bias. AI algorithms, however, can analyze vast amounts of data – including medical records, police reports, and historical claim data – to assess the validity and value of a claim objectively and consistently. This reduces the risk of inconsistencies and errors in claim valuation. For instance, AI can identify patterns indicative of fraud or exaggeration, leading to more accurate assessments and preventing fraudulent claims. Moreover, AI can expedite the assessment process by automating tasks such as image analysis (e.g., damage assessment from photos) and data comparison, allowing claims adjusters to focus on more complex cases.
Enhanced Customer Service
The integration of AI and big data is transforming customer service within the insurance industry, moving beyond traditional phone calls and emails to offer faster, more personalized, and efficient support. This enhanced approach leverages AI’s capabilities to understand customer needs, anticipate queries, and provide immediate solutions, ultimately boosting customer satisfaction and loyalty.
AI-powered solutions are streamlining interactions, allowing insurers to handle a higher volume of inquiries while simultaneously improving the quality of service. This results in reduced wait times, increased efficiency, and a more positive customer experience.
AI-Powered Chatbot Interface for Customer Inquiries
A well-designed chatbot interface for insurance companies should be intuitive and user-friendly, capable of handling a wide range of common customer inquiries. This involves integrating natural language processing (NLP) to understand the nuances of human language and machine learning (ML) to continuously improve its accuracy and responsiveness. The chatbot should be accessible through the insurer’s website and mobile app, providing 24/7 availability. The interface should offer clear navigation, allowing users to easily select their inquiry type from a menu or through natural language input. For example, a customer might type “I need to file a claim,” and the chatbot would guide them through the process, requesting necessary information and providing updates along the way. The system should also escalate complex inquiries to human agents seamlessly, ensuring a smooth transition between automated and human support.
Benefits and Challenges of AI-Powered Chatbots in Insurance
The benefits of utilizing AI-powered chatbots for customer service in the insurance industry are significant. Chatbots offer 24/7 availability, significantly reducing wait times and improving response speeds. They can handle a high volume of inquiries simultaneously, freeing up human agents to focus on more complex issues. Furthermore, chatbots can provide consistent and accurate information, reducing the risk of human error. However, challenges remain. Developing a chatbot capable of understanding the complexities of insurance policies and handling nuanced inquiries requires substantial investment in data and technology. Ensuring data privacy and security is crucial, as chatbots handle sensitive customer information. Additionally, there is a need for ongoing maintenance and updates to keep the chatbot’s knowledge base current and accurate. Finally, some customers may prefer interacting with a human agent, so a seamless handoff mechanism is essential.
Personalizing Customer Interactions and Improving Satisfaction
AI can personalize customer interactions in several ways. By analyzing customer data, insurers can tailor communication to individual needs and preferences. For instance, a chatbot can address a customer by name and refer to their specific policy details, creating a more personalized and engaging experience. AI can also proactively identify potential problems and offer solutions. For example, if a customer’s payment is overdue, the chatbot can proactively reach out and offer payment options. Personalized recommendations based on individual risk profiles can also be offered, such as suggesting additional coverage based on lifestyle changes or location. Analyzing customer feedback from interactions with the chatbot and human agents allows for continuous improvement, leading to increased customer satisfaction and loyalty. For example, if many customers are asking about a specific policy clause, the insurer can update the chatbot’s knowledge base or create FAQs to address the issue. This iterative process of improvement, driven by data analysis, is key to maximizing the effectiveness of AI in enhancing customer service.
Predictive Modeling and Analytics
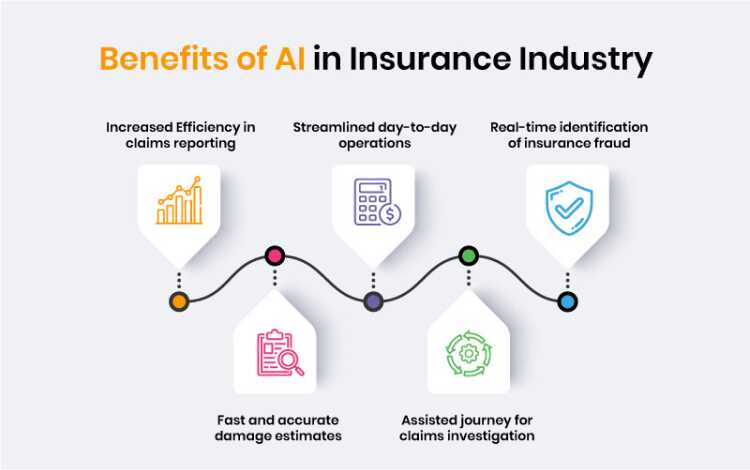
Predictive modeling is transforming the insurance industry by enabling insurers to move beyond reactive claim handling to proactive risk management. By leveraging historical data and advanced analytical techniques, insurers can forecast future claims with greater accuracy, optimize pricing strategies, and improve overall profitability. This proactive approach allows for more efficient resource allocation and a stronger competitive advantage.
Predictive modeling techniques utilize statistical algorithms and machine learning to identify patterns and relationships within vast datasets, enabling insurers to predict the likelihood of future events. These models consider numerous factors, including demographic information, historical claims data, geographic location, and even weather patterns, to generate probabilities of specific events occurring. The insights derived from these models are invaluable in informing crucial business decisions.
Types of Predictive Models in Insurance
Several types of predictive models are employed in the insurance sector, each offering unique strengths and weaknesses depending on the specific application. The choice of model often depends on the complexity of the problem, the size and quality of the data, and the desired level of accuracy.
- Generalized Linear Models (GLMs): These are widely used for their simplicity and interpretability. GLMs are particularly effective in modeling the frequency and severity of claims, allowing insurers to estimate the expected cost of future claims for specific risk profiles.
- Decision Trees and Random Forests: These techniques are well-suited for handling complex relationships between variables and can accommodate both numerical and categorical data. Decision trees visually represent the decision-making process, while random forests improve accuracy by combining multiple decision trees.
- Gradient Boosting Machines (GBMs): GBMs, such as XGBoost and LightGBM, are powerful algorithms known for their high predictive accuracy. They iteratively build an ensemble of decision trees, improving predictive performance with each iteration. They are frequently used in tasks such as fraud detection and customer churn prediction.
- Neural Networks: These complex models can capture highly non-linear relationships in data, offering potentially higher accuracy than simpler models. However, they are often more computationally intensive and require significant expertise to train and interpret effectively. Their use is increasing in areas requiring the processing of large, complex datasets, such as image recognition for assessing property damage.
Accuracy and Reliability of Predictive Models
The accuracy and reliability of predictive models vary considerably depending on the model type, data quality, and the specific application. While no model is perfect, advancements in machine learning have significantly improved predictive capabilities.
Model Type | Accuracy (Illustrative Example) | Reliability Considerations |
---|---|---|
Generalized Linear Models | 70-80% (for claim frequency prediction) | Sensitive to data outliers and assumptions about data distribution. Requires careful feature engineering. |
Decision Trees/Random Forests | 80-90% (for customer churn prediction) | Can be prone to overfitting if not properly tuned. Interpretability can be challenging with complex models. |
Gradient Boosting Machines | 85-95% (for fraud detection) | Computationally intensive. Requires careful hyperparameter tuning to avoid overfitting. |
Neural Networks | 90-98% (for image-based damage assessment) | High computational cost. Requires large datasets for training. Interpretability is often limited (“black box” nature). |
Note: The accuracy percentages provided are illustrative examples and can vary significantly depending on factors such as data quality, model complexity, and the specific application. Higher accuracy often comes at the cost of increased complexity and computational resources.
Big Data Management and Security
The insurance industry’s reliance on AI and big data necessitates robust strategies for managing and securing vast quantities of sensitive customer information. This data, encompassing everything from policy details and claims history to medical records and financial information, presents significant challenges in terms of storage, processing, and protection against unauthorized access or breaches. Effective data governance is not merely a technological undertaking; it is a critical component of maintaining customer trust and ensuring regulatory compliance.
The sheer volume, velocity, and variety of data handled by insurers demand sophisticated management systems. This includes not only efficient storage solutions but also the ability to process and analyze data quickly and accurately to support AI applications. Furthermore, the sensitive nature of much of this data necessitates robust security measures to prevent data breaches and protect customer privacy. Failure to adequately address these challenges can lead to significant financial losses, reputational damage, and legal repercussions.
Data Privacy and Compliance Regulations
The handling of personal and sensitive data in the insurance sector is heavily regulated. Insurers must adhere to a complex web of international, national, and regional regulations, such as GDPR (General Data Protection Regulation) in Europe and CCPA (California Consumer Privacy Act) in the United States. These regulations mandate specific data protection practices, including data minimization, purpose limitation, and the right of individuals to access, correct, and delete their personal data. Non-compliance can result in substantial fines and legal action. Insurers must implement comprehensive data governance frameworks that ensure ongoing compliance with these evolving regulations. This includes regular audits, employee training programs, and the implementation of technologies designed to facilitate compliance, such as data masking and anonymization techniques.
Security Measures for Protecting Customer Data
Protecting customer data requires a multi-layered security approach. This involves implementing robust technical, administrative, and physical safeguards. Technical safeguards include encryption of data both in transit and at rest, intrusion detection and prevention systems, and regular security audits and penetration testing to identify vulnerabilities. Administrative safeguards encompass policies and procedures for data access control, employee training on security best practices, and incident response plans to mitigate the impact of potential breaches. Physical safeguards involve securing data centers and physical access control to prevent unauthorized physical access to data storage devices. Furthermore, insurers should adopt a zero-trust security model, verifying every user and device before granting access to sensitive data, regardless of location. This layered approach helps to minimize the risk of data breaches and ensures the ongoing protection of customer information.
Data Breach Response and Recovery
Even with the most robust security measures in place, the possibility of a data breach remains. Therefore, a comprehensive incident response plan is crucial. This plan should Artikel clear procedures for detecting, containing, and remediating a breach, as well as for communicating with affected customers and regulatory bodies. The plan should include protocols for data recovery and business continuity to minimize disruption to operations. Regular testing and updating of the incident response plan are essential to ensure its effectiveness in the event of a real-world scenario. Post-incident analysis should also be conducted to identify areas for improvement in security measures and to prevent future breaches. A well-defined breach response plan is vital for minimizing damage and maintaining customer trust in the wake of a security incident.
Underwriting Process Optimization
The underwriting process, traditionally a manual and time-consuming task, is being significantly reshaped by the integration of AI and big data. This transformation leads to faster, more accurate risk assessments, ultimately improving efficiency and profitability for insurance companies. AI’s ability to analyze vast datasets and identify complex patterns allows for a more nuanced and data-driven approach to underwriting, moving beyond simplistic rule-based systems.
AI improves the underwriting process by automating several key tasks and enhancing decision-making capabilities. Automation reduces manual effort, minimizes human error, and frees up underwriters to focus on more complex cases requiring human judgment. Simultaneously, AI algorithms analyze data far more comprehensively than humans can, identifying subtle correlations and risk factors that might otherwise be missed. This leads to a more precise and efficient risk assessment, resulting in better pricing and reduced losses for insurers.
AI-Driven Underwriting Speed and Accuracy
AI-driven underwriting significantly surpasses traditional methods in both speed and accuracy. Traditional methods rely heavily on manual data entry, review, and analysis, a process prone to errors and delays. AI, on the other hand, can process applications and analyze data in a fraction of the time, providing near-instantaneous assessments. Furthermore, AI algorithms, trained on massive datasets, are less susceptible to biases and human error, leading to more consistent and accurate risk evaluations. For example, a study by a leading insurance technology provider showed that AI-powered underwriting reduced processing time by 70% and improved accuracy by 15% compared to traditional methods. This translates to substantial cost savings and improved customer experience.
Examples of AI in Risk Assessment
AI enhances risk assessment by leveraging various techniques. For instance, image recognition AI can analyze photos submitted for property insurance, automatically identifying potential hazards like damaged roofs or overgrown vegetation. Natural language processing (NLP) can analyze applicant data from various sources, including social media, to detect inconsistencies or red flags that might indicate higher risk. Predictive modeling, powered by machine learning, can forecast the likelihood of future claims based on a multitude of factors, allowing insurers to adjust premiums and underwriting decisions accordingly. For example, an insurer using AI to assess driver risk might incorporate telematics data (from in-car devices) to identify risky driving behaviors like speeding or harsh braking, resulting in more accurate risk profiles and personalized premiums. Another example is the use of AI in assessing the risk of fraud in health insurance claims by identifying unusual patterns in claim submissions or provider billing practices.
The Impact of IoT on Insurance
The Internet of Things (IoT) is rapidly transforming the insurance industry by providing unprecedented access to data on policyholders’ behaviors and assets. This influx of information allows insurers to develop more accurate risk assessments, personalize products, and streamline claims processes, ultimately leading to more efficient and customer-centric services. The integration of IoT data presents a significant opportunity for insurers to enhance their offerings and gain a competitive edge.
IoT devices, ranging from smart home security systems and wearable fitness trackers to telematics devices in vehicles, generate a vast amount of data that can be leveraged to improve insurance products and services. This data provides a granular view of individual risk profiles, allowing for more precise pricing and personalized risk management strategies. For example, data from a smart home security system can reveal the presence of smoke detectors, security systems, and other safety features, influencing the premium for homeowner’s insurance. Similarly, data from a telematics device can monitor driving behavior, providing insights into driving speed, acceleration, braking, and mileage, impacting auto insurance premiums.
IoT Data in Risk Assessment
IoT data significantly enhances risk assessment by providing real-time insights into policyholders’ behaviors and the condition of insured assets. For example, in auto insurance, telematics data can identify risky driving habits, such as speeding or aggressive braking, allowing insurers to offer personalized safety programs or adjust premiums accordingly. In home insurance, data from smart home devices can detect potential hazards like water leaks or gas leaks, enabling proactive interventions to prevent claims and reduce losses. This proactive approach not only benefits the insurer but also enhances the safety and security of the policyholder. For instance, a smart water leak detector could alert both the homeowner and the insurance company, allowing for rapid intervention and potentially preventing extensive water damage.
IoT Data in Claims Management
The use of IoT data streamlines claims management by providing objective evidence and accelerating the claims process. For example, in the event of a car accident, data from a telematics device can automatically record the time, location, and severity of the accident, providing crucial information for the claims adjuster. Similarly, in the case of a home insurance claim, data from smart home security cameras can provide visual evidence of the damage, speeding up the verification process and reducing the time it takes to settle the claim. This efficient claims process improves customer satisfaction and reduces operational costs for the insurer. For instance, a connected smoke detector can automatically notify the insurance company of a fire, allowing for a faster response and potentially limiting the extent of the damage.
Benefits and Challenges of Integrating IoT Data
Integrating IoT data into insurance operations offers numerous benefits, including improved risk assessment, personalized products, faster claims processing, and enhanced customer service. However, several challenges need to be addressed. These include data privacy concerns, data security risks, the potential for bias in algorithms used to analyze IoT data, and the need for robust data infrastructure to handle the large volumes of data generated by IoT devices. Furthermore, the integration of IoT data requires careful consideration of regulatory compliance and the development of transparent and ethical data usage policies. Insurers must also ensure that the use of IoT data does not discriminate against certain groups of policyholders. Successfully navigating these challenges is crucial for realizing the full potential of IoT in the insurance industry.
Future Trends and Developments
The convergence of AI, big data, and emerging technologies is poised to significantly reshape the insurance industry in the coming years. Several key trends will drive this transformation, impacting how insurers operate, interact with customers, and manage risk. Understanding these trends is crucial for insurers to remain competitive and adapt to the evolving landscape.
Three key trends will significantly shape the future of AI and big data in the insurance industry: the increasing sophistication of AI algorithms, the proliferation of alternative data sources, and the growing importance of explainable AI (XAI).
Sophistication of AI Algorithms
The ongoing development and refinement of AI algorithms, particularly deep learning and reinforcement learning, will lead to more accurate and efficient insurance processes. Deep learning models, for instance, can analyze vast datasets to identify complex patterns and relationships that traditional methods miss, leading to more precise risk assessment and fraud detection. Reinforcement learning can optimize pricing strategies and claims handling procedures in real-time, dynamically adjusting to changing market conditions and customer behavior. This increased sophistication will translate into improved underwriting decisions, more personalized products, and faster claims processing. For example, insurers are already using deep learning to analyze satellite imagery to assess property risk more accurately, considering factors like proximity to flood zones or wildfire-prone areas that traditional methods may overlook.
Proliferation of Alternative Data Sources
Beyond traditional data sources like credit scores and driving records, insurers are increasingly leveraging alternative data sources, including telematics data, social media activity, and IoT sensor data. This broader data landscape allows for a more holistic understanding of risk and customer behavior, leading to more accurate risk profiling and personalized insurance offerings. For instance, telematics data from connected cars can provide real-time insights into driving habits, enabling insurers to offer usage-based insurance (UBI) with premiums adjusted based on individual driving behavior. Similarly, analyzing social media data can provide insights into lifestyle choices and risk factors, allowing for more targeted marketing and product development.
Growing Importance of Explainable AI (XAI)
As AI plays a larger role in insurance decision-making, the need for transparency and explainability increases. Explainable AI (XAI) focuses on developing AI models that can provide clear and understandable explanations for their predictions. This is crucial for building trust with customers and regulators, ensuring fairness and preventing bias in insurance decisions. For example, if an AI model denies a claim, XAI techniques can provide a detailed explanation of the factors that led to the denial, ensuring transparency and addressing potential concerns about algorithmic bias. The demand for XAI is expected to grow significantly as regulatory scrutiny of AI in finance increases.
Timeline of AI and Big Data Evolution in Insurance (Next 5 Years)
The following timeline illustrates the projected evolution of AI and big data applications within the insurance sector over the next five years:
Year | Expected Developments | Examples |
---|---|---|
2024 | Widespread adoption of AI-powered chatbots for customer service; increased use of AI in fraud detection; initial implementation of XAI in some areas. | Many insurers already use chatbots, but expect more sophisticated, AI-driven versions. Fraud detection will become more proactive, identifying patterns before they lead to losses. Early XAI implementations will focus on simple decisions. |
2025 | Expansion of UBI programs; wider adoption of AI-powered risk assessment models; increased focus on data privacy and security. | More insurers will offer UBI based on diverse telematics data. Risk assessment will become more granular, incorporating alternative data sources. Data privacy regulations will drive investment in secure data management. |
2026 | Mature XAI solutions implemented across multiple insurance processes; development of AI-driven personalized insurance products; further integration of IoT data. | XAI will become a standard feature in many AI systems, enhancing transparency and trust. Insurers will offer highly customized products based on individual risk profiles. IoT data will be more seamlessly integrated into risk assessment and claims handling. |
2027 | AI-driven predictive maintenance for property insurance; advanced analytics for personalized claims management; greater use of blockchain for secure data sharing. | AI will predict potential property damage and proactively suggest preventative measures. Claims processing will become more efficient and personalized, offering tailored solutions. Blockchain will improve data security and interoperability between insurers. |
2028 | AI-powered underwriting becomes the norm; fully automated claims processing for simple claims; development of sophisticated AI models for complex risk assessment (e.g., climate change risk). | Most underwriting decisions will be AI-driven, significantly speeding up the process. Simple claims will be processed automatically, reducing human intervention. Advanced AI will model the impact of climate change on insurance risk. |
Q&A
What are the biggest challenges in implementing AI in insurance?
Significant challenges include data security and privacy concerns, the need for substantial investment in infrastructure and skilled personnel, and the ethical implications of using AI for risk assessment.
How does AI improve customer service in insurance?
AI-powered chatbots provide 24/7 support, answer common queries instantly, and personalize interactions based on individual customer data, leading to improved response times and higher customer satisfaction.
Will AI replace human jobs in the insurance industry?
While AI automates certain tasks, it’s more likely to augment human capabilities. Human expertise will remain crucial for complex decision-making, customer relationship management, and ethical considerations.
What are the regulatory implications of using AI in insurance?
Insurers must comply with data privacy regulations (like GDPR and CCPA) and ensure fairness and transparency in AI algorithms to avoid bias and discrimination.